Source: China Superhard Materials Network
Abstract: Using the macroscopic hardness model based on database and data transmission combined with machine learning, we can predict the Vickers hardness (Hv) of various crystal materials. A team led by Professor Eva Zurek from the Department of Chemistry at the State University of New York at Buffalo, through AFLOW...
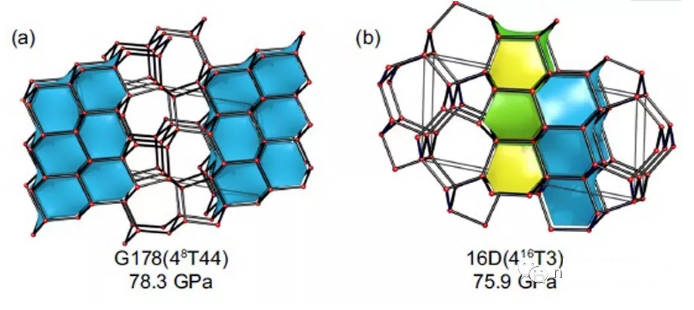
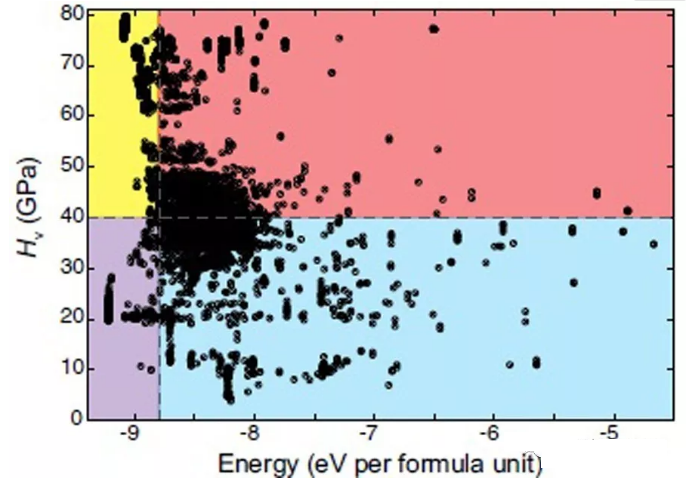
Using the macroscopic hardness model based on database and data transfer combined with machine learning, the Vickers hardness (Hv) of a variety of crystalline materials can be predicted. A team led by Professor Eva Zurek from the Department of Chemistry at the State University of New York at Buffalo obtained a linear relationship between Hv and shear modulus through the RESTful interface in AFLOW and calculated the Vickers hardness Hv. The predicted results are in good agreement with the results of the first principle calculation and agree well with the experimental results. These techniques allow one to quickly calculate the reasonable hardness values for a given crystal structure based on the elastic properties of machine learning, and can use these predicted hardness values to calculate the fit of each superhard phase. This technique is implemented in an Evolutionary Algorithm (EA) and then applied to the carbon system to find stable and superhard phases. In their search, 79 different topologies with stable kinetics, low energy, and Hv > 40 GPa were found, of which 43 topologies have not been reported before. One is expected to find cheap superhard carbon materials to replace expensive diamonds, which was recently published in npj Computational Materials 5:89 (2019).
The study found that there is good agreement between the experimental Vickers hardness (Hv) of various materials and the hardness calculated by the three macro hardness models. Shear and/or moduli are the main characteristics of the three macro hardness models. The parameters are obtained by the following two methods: i) the first-principles calculation model of the flow-AEL (flow automatic elastic library), and the second, the material data in the flow database is used as a sample, and the machine learning (ml) model is trained. Quickly estimate Hv ml values, which can be used in conjunction with evolutionary search to predict stable superhard materials. This method is implemented in the X Tal OPT evolution algorithm. Each crystal is minimized to the nearest local minimum, Its Vickers hardness is calculated from the linear relationship of the Trent shear modulus. Both energy/焓 and Hv ml are used to determine the fit of the structure. The method was applied to the carbon system and found 43 A new superhard phase. Topological analysis shows that the predicted structure is slightly stronger than the diamond, which contains a large amount of diamond and/or hexagonal carbon structure.
-All diamond tools can be ordered and fabricated!
-Henry Wang | Quote Manager
-WhatsApp:+86-13459035657
-Email: ceo@zdiamondtools.com
-Web www.zdiamondtools.com
Company Profile
Certifications
Company Team
Exhibition
Logistics
FAQ